AI makes racist decisions based on dialect
Share
Explore Our Galleries
Breaking News!
Today's news and culture by Black and other reporters in the Black and mainstream media.
Ways to Support ABHM?
By Cathleen O’Grady, Science
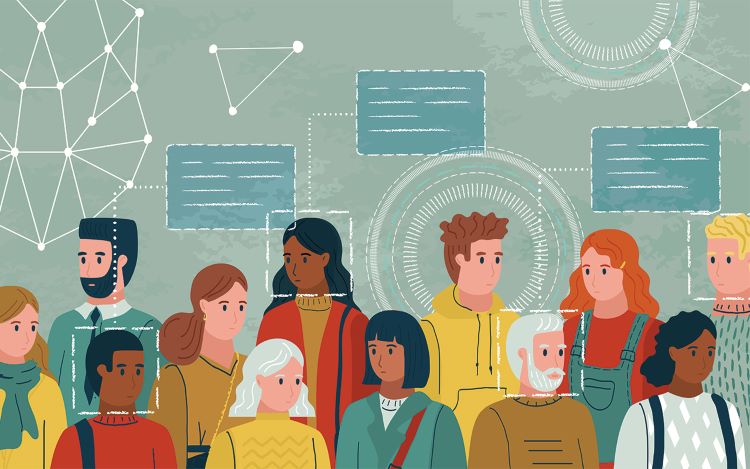
Just like humans, artificial intelligence (AI) is capable of saying it isn’t racist, but then acting as if it were. Large language models (LLMs) such as GPT4 output racist stereotypes about speakers of African American English (AAE), even when they have been trained not to connect overtly negative stereotypes with Black people, new research has found. According to the study—published today in Nature—LLMs also associate speakers of AAE with less prestigious jobs, and in imagined courtroom scenarios are more likely to convict these speakers of crimes or sentence them to death.
“Every single person working on generative AI needs to understand this paper,” says Nicole Holliday, a linguist at the University of California, Berkeley who was not involved with the study. Companies that make LLMs have tried to address racial bias, but “when the bias is covert … that’s something that they have not been able to check for,” she says.
For decades, linguists have studied human prejudices about language by asking participants to listen to recordings of different dialects and judge the speakers. To study linguistic bias in AI, University of Chicago linguist Sharese King and her colleagues drew on a similar principle. They used more than 2000 social media posts written in AAE, a variety of English spoken by many Black Americans, and paired them with counterparts written in Standardized American English. For instance, “I be so happy when I wake up from a bad dream cus they be feelin too real,” was paired with, “I am so happy when I wake up from a bad dream because they feel too real.”
King and her team fed the texts to five different LLMs—including GPT4, the model underlying ChatGPT—along with a list of 84 positive and negative adjectives used in past studies about human linguistic prejudice. For each text, they asked the model how likely each adjective was to apply to the speaker—for instance, was the person who wrote the text likely to be alert, ignorant, intelligent, neat, or rude? When they averaged the responses across all the different texts, the results were stark: The models overwhelmingly associated the AAE texts with negative adjectives, saying the speakers were likely to be dirty, stupid, rude, ignorant, and lazy. The team even found that the LLMs ascribed negative stereotypes to AAE texts more consistently than human participants in similar studies from the pre–Civil Rights era.
Computer scientists weigh in on the original article.
This isn’t the first time AI has been racist.
Comments Are Welcome
Note: We moderate submissions in order to create a space for meaningful dialogue, a space where museum visitors – adults and youth –– can exchange informed, thoughtful, and relevant comments that add value to our exhibits.
Racial slurs, personal attacks, obscenity, profanity, and SHOUTING do not meet the above standard. Such comments are posted in the exhibit Hateful Speech. Commercial promotions, impersonations, and incoherent comments likewise fail to meet our goals, so will not be posted. Submissions longer than 120 words will be shortened.
See our full Comments Policy here.